AI IoT, Cyber Physical System
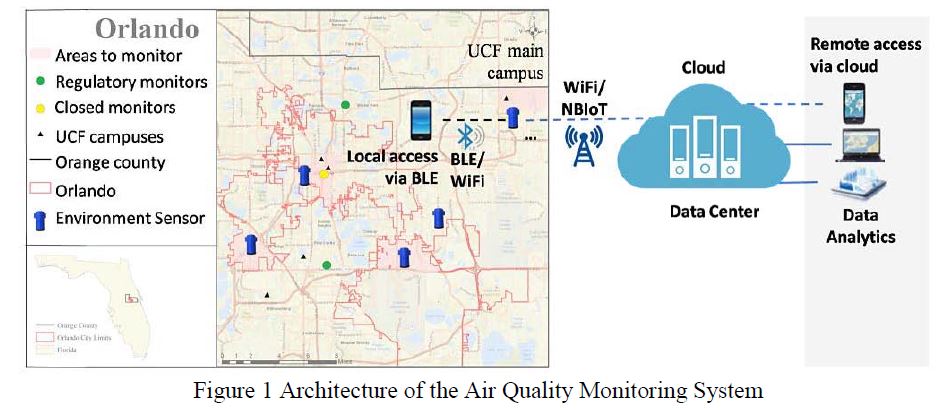
Project Developed Wireless Air Quality Sensor Network
STAIR: Smart Air Network: http://3.85.149.13/map
A Secure, Trustworthy, and Reliable Air Quality Monitoring System for Smart and Connected Communities
A critical application of smart technologies is a smart, connected, and secured environmental monitoring network that can help administrators and researchers find better ways to incorporate evidence and data into public decision-making related to the environment. In this project, the investigators will establish a secure, trustworthy and reliable air quality monitoring network system using densely deployed low-cost sensors in and around the city of Orlando, Florida, to better inform development of pollution mitigation strategies in the region. Access to the urban-scale air quality sensor data and forecasts can have a positive social impact on environmental justice, public health, and sustainability initiatives. The investigators will incorporate the outcome of the project into courses on computer and network security and privacy, mobile computing, environmental sciences and engineering, and social science. The proposed work will provide hands-on exercises, research, and educational opportunities for undergraduate, graduate students and K-12 students.
The objectives of this project include performing remote low-cost sensor calibration, drift and malfunction detection. An innovative modeling method will be developed to perform remote calibration for low-cost PM2.5 sensors. A triple-sensor system will be developed, employing an operational statistical method that cross-evaluates sensor measurement data every hour to identify potential sensor drifts and malfunctions. The project team will build a trustworthy air quality monitoring network. A trusted boot strategy will be developed to ensure the sensor firmware is genuine at bootstrapping, performing dynamic analysis of states of the system, sending the measurement to a verifier for remote attestation, and accepting commands from the verifier to act on violations. The team will also create an accurate deep learning-based air quality prediction system based on a novel two-stage semi-supervised learning framework from noisy and mixed-labeled sensor big data. Social scientists on the team will conduct a social behavioral study of air quality monitoring and prediction. This project emphasizes sustainable empowerment of residents through processes of education on air quality and training on data utilization and advocacy. The project goes beyond passive citizen science to enable citizens to become advocates for their interests to increase not only outside air quality but also the overall quality of life of citizens in the community.
- [CVPR’20] Adnan Siraj Rakin, Zhezhi He and Deliang Fan, “TBT: Targeted Neural Network Attack with Bit Trojan,” 2020 IEEE/CVF Conference on Computer Vision and Pattern Recognition (CVPR), June 16-18, 2020, Seattle, Washington, USA
- [CVPR’20] Zhezhi He,Adnan Siraj Rakin, Jingtao Li, Chaitali Chakrabarti and Deliang Fan, “Defending and Harnessing the Bit-Flip based Adversarial Weight Attack,” 2020 IEEE/CVF Conference on Computer Vision and Pattern Recognition (CVPR), June 16-18, 2020, Seattle, Washington, USA
- [AAAI’20] Li Yang, Zhezhi He and Deliang Fan, “Harmonious Coexistence of Structured Weight Pruning and Ternarization for Deep Neural Networks,” Thirty-Fourth AAAI Conference on Artificial Intelligence (AAAI), Feb. 7-12 2020, New York, USA (spotlight)
- [DAC’20] Li Yang, Zhezhi He, Yu Cao and Deliang Fan. “Non-uniform DNN Structured Subnets Sampling for Dynamic Inference”. In: 57th Design Automation Conference (DAC), San Francisco, CA, July 19-23, 2020